— 7 min read
Unlocking the Power of Construction Data Analytics: Insights for Better Decisions
Last Updated Oct 30, 2024
Last Updated Oct 30, 2024
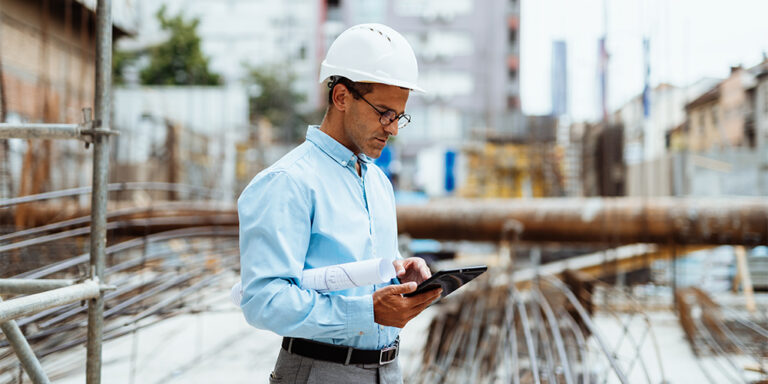
In today’s construction industry, data is being collected daily—on phones, on computers and tablets, by cameras and even on some of the devices used to perform construction work. If a general contractor (GC) isn’t monitoring and leveraging that information by using data analytics, they’re missing out on critical information—and may be making decisions that are less informed than they should be.
Data analytics can be defined as the process of examining data to uncover patterns, draw conclusions and identify actionable insights. Analytics provide information and feedback on how well construction companies are performing across various projects, tasks, and processes.
Though some elements of data analytics can be complex, there are also simple and relatively straightforward ways a GC can implement this process into their business—and make sure their choices are backed by reliable data.
From basic compliance measures, to descriptive statistics that provide a clear picture of a project’s health throughout its lifecycle, data gives organizations insight into what’s working and where to focus their attention for improvement on projects.
This article explores the efficacy of data analytics in the construction industry, including practical steps for implementing a system of data analysis, challenges with acquiring data, and best practices for fostering a data-driven culture on any construction team.
Table of contents
Key Types of Data Dashboards for Construction
Data analytics can tell stories of the past and present state of work and , and predict future opportunities and issues.
The following depicts a few key ways these two types of analyses can make a GC’s job easier. These methods often form the basis for further analysis, involving looking over data that’s already been collected and stored to identify trends and patterns.
Descriptive Analytics
Descriptive analytics involves programs that describe the current state of work. Descriptive statistics can provide a clear, straightforward overview of past and current activities in real time, making it easier to identify whether progress deadlines are being met.
One easy way to start implementing descriptive analytics is with basic compliance measures. A company can establish and track key performance indicators (KPIs) that reflect adherence to safety protocol and regulatory standards. These basic compliance metrics provide a straightforward and data-driven overview of current operations, making it easier for project leadership to identify any areas that may need attention.
The business side of analytics basically means putting a measure to performance and giving real-time information or real-time feedback on how we are performing on any given project, task or process.
Justin Loehn
Analytics Engineer
RNGD
Predictive Analytics
Predictive analytics is a more advanced and sophisticated form of data analysis, and is a helpful tool for GCs and project leadership since these programs can anticipate future issues and costs using statistical modeling. This can be useful in project planning, resource allocation and risk management.
There are many varieties of predictive analytics techniques, including regression analysis and clustering. In general, these tools and models use historical data to forecast future outcomes. For example, using clustering models, GCs can categorize materials based on similarities among customer material requests and historical pricings to compute costs for future projects —using all of this information to generate a conceptual bid estimate.
We might create a cluster, for example, for cabinetry materials—which would include screw sets and drawer slides. We would use these groupings to determine how we’ve bid in the past on the same items and then estimate an average price point.
Then we can generate a conceptual estimate, providing a good starting point for the bid. And by using factor analysis, we eliminate the need for estimators to manually dig through all the data; the factor analysis will automatically group these items together.
Justin Loehn
Analytics Engineer
RNGD
Practical Implementation of Data Analysis
One of the most vital parts of analyzing data is making sure the data is useful to the viewer. Construction companies have to make sure they’re competently collecting data that can best inform the KPIs that they have identified for themselves.
The principle of "garbage in, garbage out" applies here—if data collected is poor, imprecise or inaccurate, any insights derived from it will be just as flawed. By implementing standardized data collection practices, companies can make sure that the information they analyze is both reliable and actionable.
Equally important is the training and adoption of these standardized practices. Team members must be educated on good data habits, and trained to accurately review visualizations and regularly look at relevant data.
Challenges of Data Analytics
Getting Company Buy-in
The main challenge to data analytics is getting buy-in. Some team members, especially those used to more traditional methods of assessing progress and cost, may be resistant to change.
To overcome this, demonstrate how data analysis can provide tangible improvements little by little. Starting with tools that are proven to increase efficiency or cut costs can help illustrate the value of these programs and encourage wider adoption.
Navigating Costs
Another significant challenge is the cost associated with implementing data analytics. From purchasing software and tools to hiring skilled personnel, expenses can add up quickly. Advanced analytics platforms, data storage solutions and visualization tools like Power BI, Tableau, or Looker often come with substantial licensing and subscription fees.
Additionally, hiring or training staff to manage and analyze data—such as data analysts, engineers, and scientists—may also be necessary to budget for in order to successfully develop a data program.
Companies can mitigate these costs by starting with the basics. Implementing fundamental data collection and analysis practices can provide immediate benefits and lay the groundwork for more advanced analytics in the future. This incremental approach allows companies to build their data capabilities over time without incurring prohibitive costs upfront.
Best Practices for Implementing Data Analytics
Data analytics has the potential to transform construction operations, driving informed decision-making and improved project outcomes. To fully realize these benefits, it's important to implement data analytics thoughtfully and strategically.
Build a data-driven culture.
The evolution of technology presents new opportunities to enhance efficiency. Finding success in data analytics relies on the solid foundation of a data-driven culture, and a willingness to change old methods from every member of the crew.
Company leadership can start by emphasizing the importance of good data habits. Regularly reviewing visualizations and reports generated by data analytics tools encourages team members to understand their value, and how to use and read them correctly. Before analytics can be most useful, an environment must be fostered where data is valued and utilized effectively.
Adopt tools incrementally.
It’s perfectly OK — and in some cases, best — to go slow with data analytics tools. While there are dozens of programs and tools that hold the capacity to forecast costs, automate schedules and predict obstacles, adopting tools incrementally can make sure that involvement with data analytics grows at the same rate as buy-in and comfort level with increasing technology.
It's often effective to start with one team at a time, allowing for focused training and smoother adoption. Prioritizing which data sets to start with can also provide quick wins and demonstrate the value of analytics early on.
Work with a Data Process Analyst.
There are specialists who have spent their careers helping companies develop their data processes, and hiring a data process analyst can be a game-changer in gaining buy-in across a company, as well as training team members to successfully collect good data.
A data process analyst can guide teams through the initial setup of data collection and analysis practices, helping them understand how these tools work and how they can help them at their jobs. They can also provide ongoing support and training.
The Future of Data Analytics in Construction
As the industry evolves, there’s likely to be a significant shift from merely descriptive analytics to more advanced predictive and prescriptive analytics — tools and processes that can forecast future obstacles and suggest actionable and proactive steps to handle them.
The field of construction is on the cusp of a data-driven transformation, where Artificial intelligence (AI) and Machine Learning (ML) are set to play pivotal roles. These technologies can analyze vast amounts of data far more quickly than human capabilities. Machine learning algorithms can enhance predictive analytics by continuously learning from new data, and improving their accuracy over time. The application of AI and ML in construction could range from optimizing project schedules and resource allocation to predicting equipment failures and improving safety measures.
But in the current state of the industry, most companies don’t have data that’s mature enough to make these tools effective. Many are still in the early stages of their data analytics adoption journeys, and should focus for now on improving data quality, standardizing their data intake process and training team members to understand and use data-driven reports., standardizing their data formats and training team members to understand and use data tools.
Was this article helpful?
Thank you for your submission.
0%
0%
You voted that this article was . Was this a mistake? If so, change your vote here.
Scroll less, learn more about construction.
Subscribe to The Blueprint, Procore’s construction newsletter, to get content from industry experts delivered straight to your inbox.
By clicking this button, you agree to our Privacy Notice and Terms of Service.
Categories:
Tags:
Written by
Justin Loehn
Justin Loehn is a dynamic professional with a background in data analytics, data engineering, and process analysis, currently contributing his expertise to RNGD. With over a decade of experience in Business Analytics and Market Research Analytics, Justin brings a wealth of knowledge to his role, having transitioned to the construction industry two years ago. Born and raised in New Orleans, Justin graduated from Brother Martin High School and holds an MBA from Loyola University of New Orleans. His career path has been marked by a commitment to leveraging data-driven insights to revolutionize operational efficiencies and strategic decision-making in a variety of business sectors. At RNGD, Justin applies his fresh perspective and analytical prowess to drive innovation in managerial processes through automation and advanced data techniques. Justin's role is pivotal in transforming how RNGD approaches projects, ensuring that stakeholders benefit from his detailed analytical reports, which enable informed, data-driven decisions. His aspiration is to continue enhancing RNGD's capabilities within the construction sector, pushing the boundaries of what's possible through technology and strategic business solutions. Outside of work, Justin is deeply rooted in the local community, actively engaging in initiatives that contribute to the growth and development of New Orleans. With his blend of analytical acumen and a passion for the construction industry, Justin Loehn embodies the spirit of innovation and progress in his professional journey at RNGD.
View profileTrey Strange
19 articles
Trey Strange is a Peabody and Emmy-Award winning writer and producer based in Brooklyn, NY. Throughout his career, Trey has worked for the Huffington Post, Houston Chronicle, Out Magazine, Brooklyn Eagle, CNBC, INTO, and New York Magazine's Bedford + Bowery. He received his Masters in Journalism and Middle East studies from New York University, and Bachelors in the same subjects from the University of Houston.
View profileExplore more helpful resources
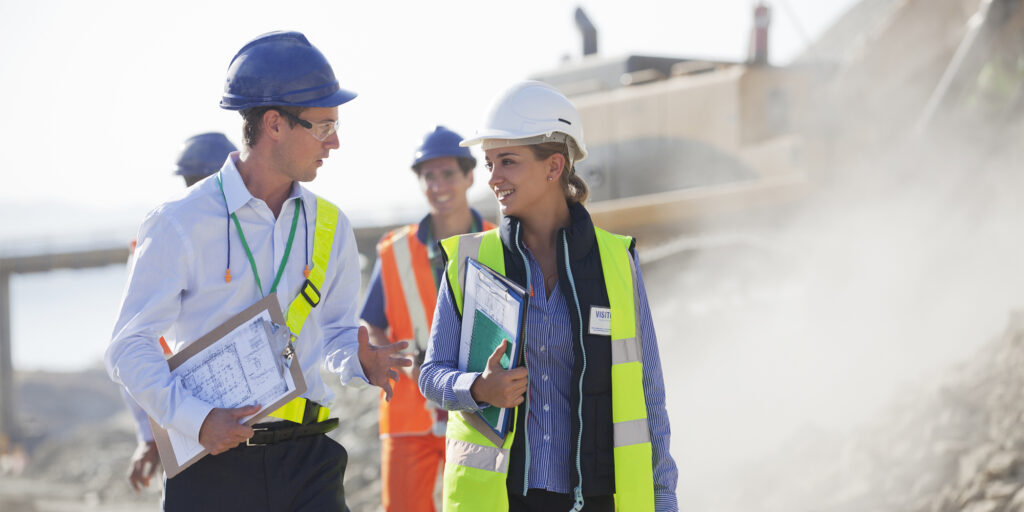
Building Together: How Integrated Project Delivery Creates Shared Value
As technology and innovative processes continue to evolve the construction industry, the need for openness and collaboration among teams and stakeholders has increased significantly. Traditional project delivery methods, such as...
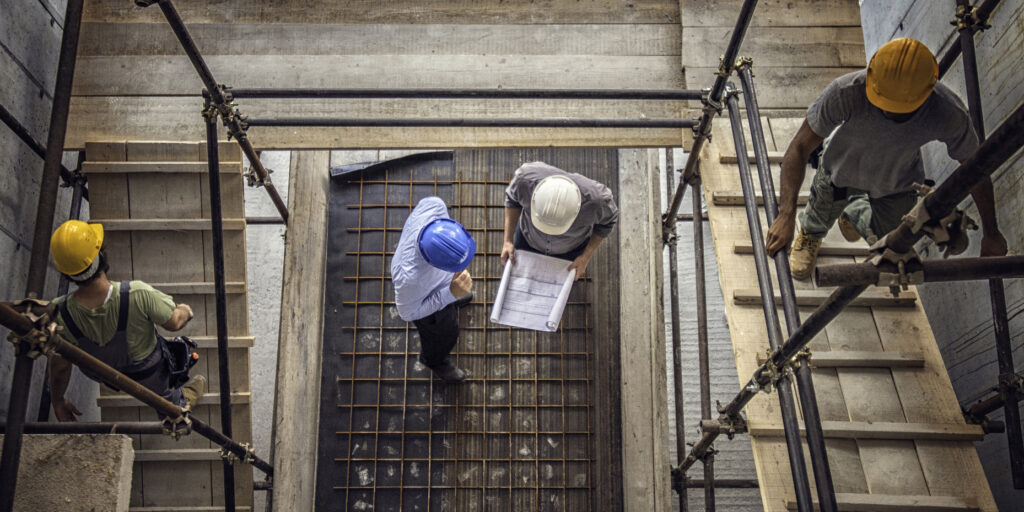
Construction Collaboration: Navigating the Intersection of Project Management and Accounting
Accounting and project teams work together to move the financial aspects of projects through to completion. Data sharing allows for concurrent review to make this possible. Teams need to share...
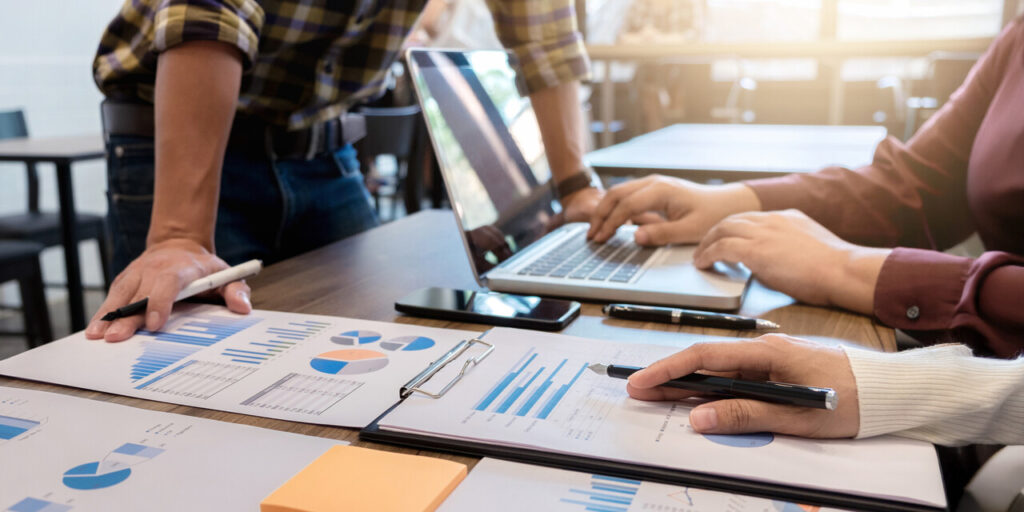
5 Reasons to Separate Project and Business Financials in Construction
Like most things in construction, the industry has a unique challenge that most ERPs don’t account for: tracking financial health of multiple projects individually, while still understanding the financial health...
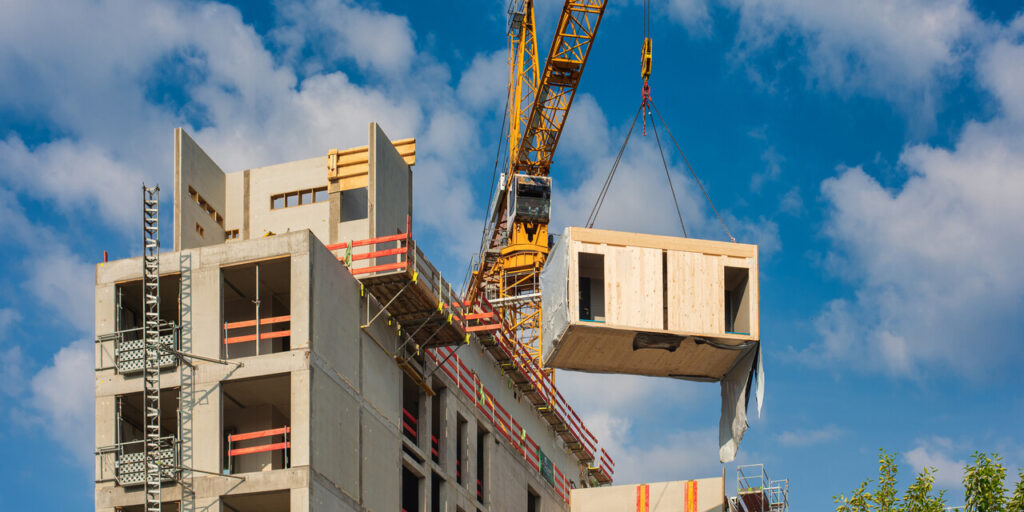
Modular Construction: Assembling the Future of Construction
As the construction industry deals with a number of converging challenges, modular construction is emerging as a forward-thinking and highly technical solution to streamline the building process. Modular construction is...